Merry Catsmas: BTW, I Still Don't Get Agentic AI
This is a small rant about the new (not so) hotness of Agentic AI. You're welcome.
Firstly, Happy Christmas / Hanukkah / Kwanzaa / Boxing Day / Bodhi Day for all who celebrate these things around this general time of the year. I do not, and so to me, this is merely a very subdued Wednesday when nothing is open. But for the sake of merriment, here’s a picture of a Christmas Catsmas card I shared with my parents years ago. This is technically a wedding photo.1 David (aka, Husbot) was possibly re-evaluating his life choices at that point, but by then, it was too late.
Before anyone calls the ASPCA, no actual cats were harmed in the making of this photo/Catsmas card. The only thing suffering here is my dignity.
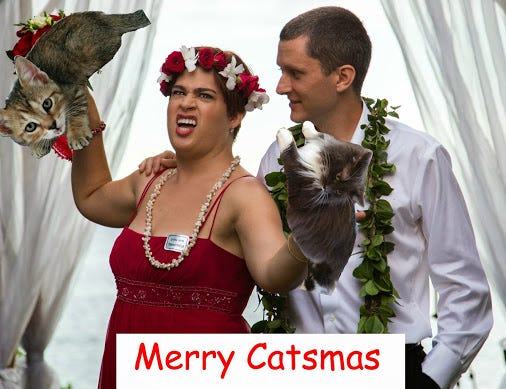
Now then, let’s talk about what inspired this post — me spending a few hours learning about agentic AI!
Carey Tries to Learn Things
So, a few days ago, I came across this Deeplearning.ai course on ‘Multi AI Agent Systems with CrewAI’. I’d like to start out with some kudos before I dig in though:
Deeplearning.ai is doing a lot to make all these AI thingies very accessible to regular folks who are curious but lack a CS degree. The courses are almost always free, interactive, and interesting, and this post is not a dunk on them or the platform.
This is also not a dunk on CrewAI, who also does a good job sharing their tools and how to actually use those tools. The tooling is free and open source, and you can install it locally on any computer. The documentation is reasonably good, and they have an active developer community. They even have gobs of free templates and examples to play with. You should play with it, notwithstanding my critique. Who knows, you may find value here.
So yeah, overall, it was an informative way to burn a few hours and learn about agentic AI. Now, some of you may be asking WTF agentic AI is, so I’ll explain.
Agentic AI refers to AI systems that are capable of independent action and decision-making/goal achievement. They use planning, task assignment, and orchestration to solve complex, multi-step problems (like, say, researching, writing, and editing a blog post, finding and booking a venue for an upcoming party, or acting as virtual assistants). They’re different from generative AI/chatbots in that while they carry out a task or tasks defined by a human, they do not need the human’s assistance to carry out those tasks. A properly functioning agentic system is supposed to figure out a solution on its own when it gets stuck, and creatively problem solve when faced with a blocker.
Agentic AI is also supposed to be a bit more robust, adaptable, and creative than robotic process automation (RPA), in that it doesn’t require as much structure and pre-planning to get the computer to make decisions. RPA systems are not intuitively adaptable — if your web scraping RPA breaks because the URL of the website you’re scraping 404s, you’re gonna have to fix that manually. Agentic AI has some self-healing/adaptation abilities to work around those types of issues.
Here’s a table showing the differences between LLMs/Chatbots, Agentic AI, and RPA. I had ChatGPT whip up the initial table and then I modified some of the language & examples because ChatGPT wasn’t as clear about Agentic AI as I would have liked.2 I am also deeply, deeply skeptical about claiming that AI agents are “autonomous”.
With that out of the way, let’s get to the ranting.
I mentioned above that when it’s done right, agentic AI is better than Chatbots/LLMs and RPA. It’s the hotter, smarter, more charismatic sibling of the AI programming world. It’s Marcia Brady from the Brady Bunch, essentially.3
But actually playing with these tools never seems to justify the hype. I don’t think this is unique to CrewAI — I have dabbled with other agentic AI tools like AutoGPT and Claude 3.5 Sonnet Computer Use before, and they have also let me down. But I just didn’t exactly know how to articulate why. Somehow, going through the Deeplearning/CrewAI training series has given me enough insight to explain why …
Agentic AI is Mostly Over-Hyped Bullshit
Yeah. So, I have seen a bazillion folks touting agentic AI lately. Especially “AI experts” on LinkedIn. Oh God. So many “AI Experts” on LinkedIn. That made the Deeplearning/CrewAI course + the holiday a perfectly good reason to actually learn more.
Plus, CrewAI has a number of interesting examples it shared during the training course, including a research & writing example I was excited to play with, a multi-agent collaboration agent for financial analysis, and a tailored resume creator. Within the confines of the training environment, the individual agents ran reasonably well. But if you try to export them out of that environment (to say, a local machine), things break in really dumb, inexplicable ways.
For example, CrewAI (the platform) creates a local database for storing past agent runs and prompts. This is touted as a way to save time, compute, and tokens when running agents. Essentially, the agents can refer back and remember what they’ve done in the past, which is good.
However, what happens in practice is that the whole system gets easily confused if you run more than one agent on your local system, for example, if you run the finance agent, and then the resume agent, and then research agent. Not chained together as a single agent, mind you. Independently.
This is because everything agent’s output gets stored in the same database, with no differentiation, and this causes future agents to do goofy shit like respond to a research task about ‘Machine Unlearning’ with a dump of a mock resume stored during the previously-run resume agent task:
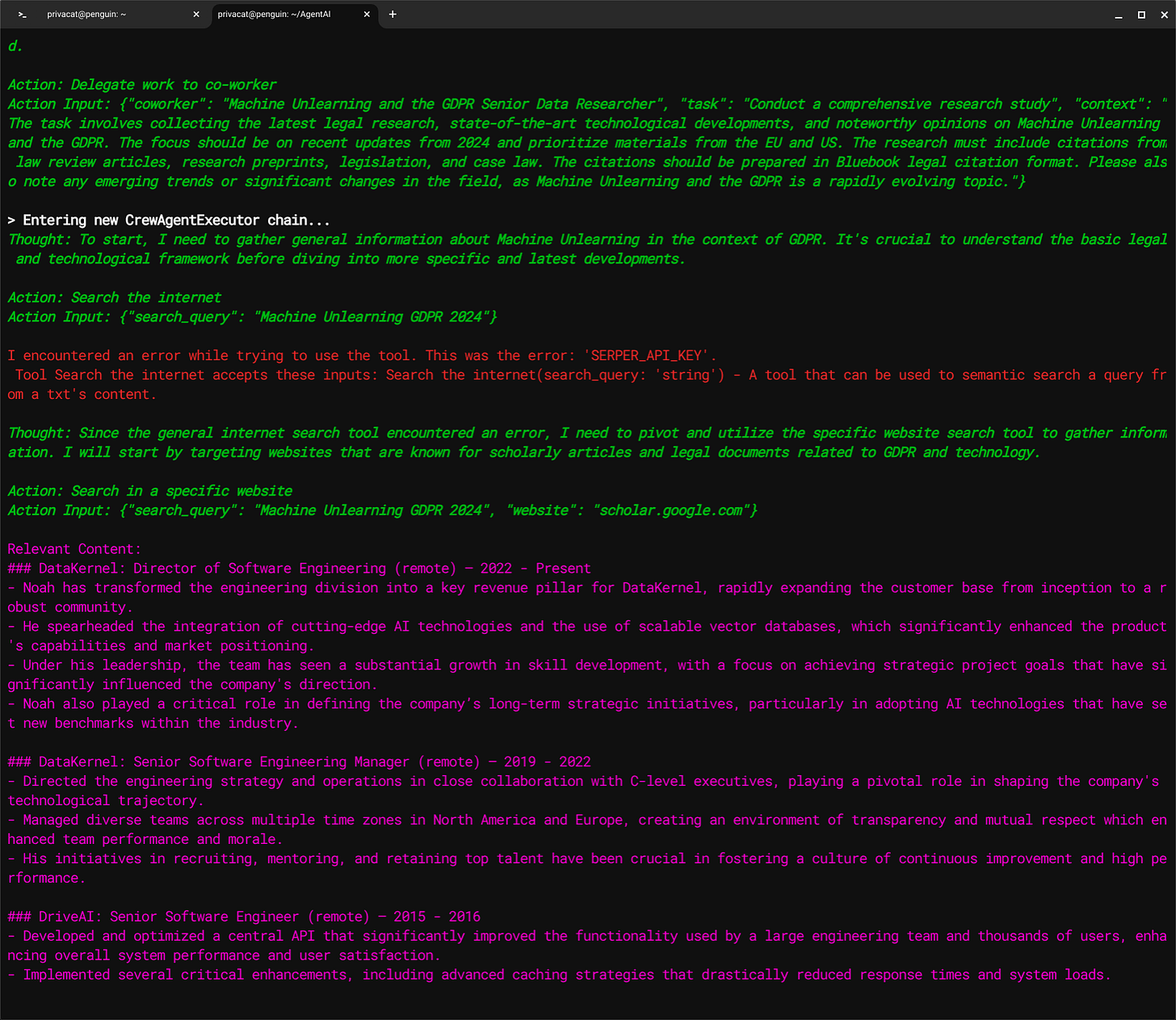
Similarly, agents would often just get caught in a loop, ‘researching’ the same URL with the same search query over, and over, and over again, helpfully and verbosely reporting that there was an error, but never figuring out how to work around that error.
Even when the program delivered something that was vaguely adjacent to my request, it was … beige and lifeless. I’m talking ChatGPT 3.5 levels of stale text. I mean, these are just words. There is zero substance here.
**Introduction**
In the rapidly evolving world of data privacy, the concept of 'Machine Unlearning' is emerging as a key player in compliance strategies. This blog seeks to explore the definition of Machine Unlearning, examine its applications, and discuss the compliance with key regulations such as GDPR and CCPA. We'll also delve into the technological and legal challenges associated with Machine Unlearning, future trends, and the importance of integrating these practices into existing legal frameworks.
**What is Machine Unlearning?**
Machine Unlearning refers to the proactive process of removing learned data from machine learning models. This process ensures that the data is not merely deleted superficially but is actually forgotten by the algorithms, thus enhancing data privacy. This concept is particularly relevant in an era where data deletion requests by users have become frequent under regulations like GDPR.
**Applications and Compliance**
Practically, Machine Unlearning helps organizations adhere to data privacy laws by ensuring that any right to erasure request is fully honored not just in visible data storage but also in complex machine learning models. For instance, under GDPR and CCPA, users can request the deletion of their data. Machine Unlearning ensures that these deletions are reflected in the predictions and operations of AI systems, thus maintaining compliance.
**Technological and Legal Challenges**
The implementation of Machine Unlearning is not without challenges. Technologically, retraining models to forget data can be resource-intensive and complicated. Legally, the ambiguity in data privacy laws about the extent of 'forgetting' data can create compliance challenges. Organizations must navigate these waters carefully, balancing technological limitations with legal expectations.
**Future Trends**
The future of Machine Unlearning is promising but requires more robust frameworks. As AI continues to permeate various sectors, the demand for mechanisms to ensure 'data forgetfulness' will likely increase. Innovations in Machine Unlearning will be crucial in managing these demands effectively.
**Conclusion: Integrating Machine Unlearning into Legal Frameworks**
As we look towards the future, integrating Machine Unlearning into legal and regulatory frameworks will be essential. This will not only support compliance with data privacy laws but also foster trust in AI systems. Stakeholders must work together to ensure that the legal provisions keep pace with technological advancements, paving the way for a privacy-respectful AI-driven world.
This blog post is designed to be informative and engaging, providing clear insights into the complex topic of Machine Unlearning and its significance in the current data privacy landscape.
Now, I’m not a coder. It’s possible that despite my reasonably decent understanding of how to get LLMs, systems, and Husbots to generate outputs that I want, that I nonetheless just don’t get agentic AI.
Alternatively, it’s possible (as David surmised) that CrewAI hasn’t quite gotten all the bugs out (though again, I’ve seen similar failures on AutoGPT and with Claude). Finally, it’s possible that agentic AI just isn’t designed to do the kinds of things I intuitively think it should be able to do with a little structured assistance.
It's just weird to me that these systems are talked up as being the solution to everything big and difficult, when it doesn't look like they do the complicated stuff very well at all. Sure, they’re slightly better than RPA, but you don't need to be paying ChatGPT-level costs or burning resources to do RPA-level tasks.
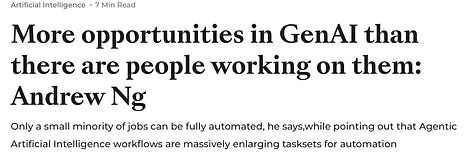
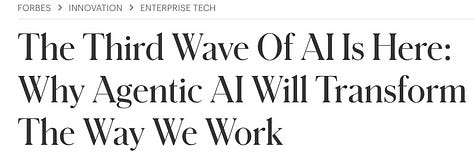
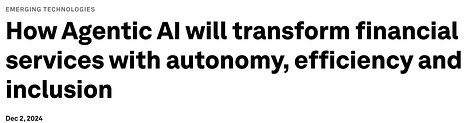
As I said at the beginning, none of this is to blast CrewAI specifically. I still just don’t see what all the hype is about. Maybe someday there will be a good use-case that makes it all worth the hype, but right now, I’ll go back to ignoring all the LinkedIn experts claiming that this is the next AI messiah destined to take all our jobs and just keep writing and researching on my own, thanks.
Maybe someone can tell me what I’m missing in the comments.
PS: Here’s a picture of what I’m reading right now: The excellent ‘AI Act Compact’ by the brilliant Tea Mustać and Peter Hense. This book is so interesting, that this very scared, adoptable, smol kitten is gracing me with his presence while I read it. This is grade-A, kitten-approved content, y’all.
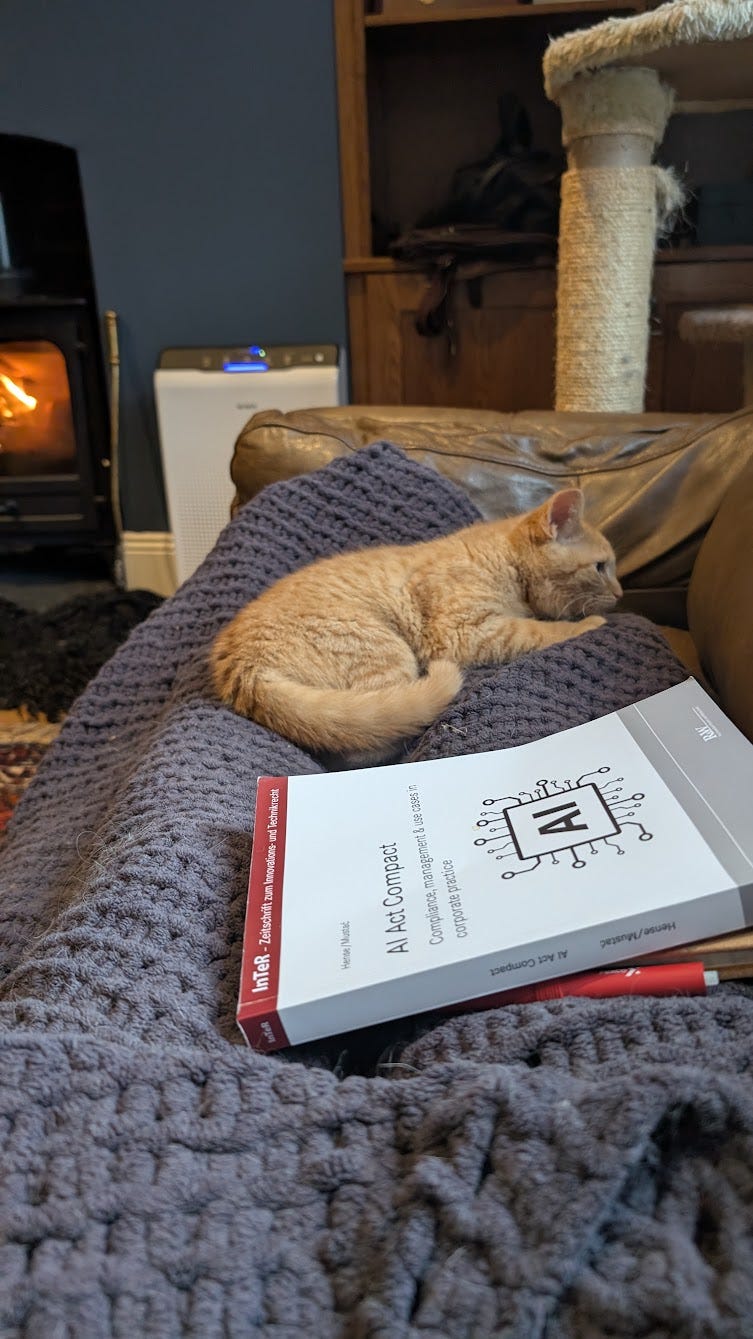
This was at the end of the shoot, and I was told to be goofy/ragey. I do not always look like this.
Feel free to pause and reflect on that.
For what it’s worth, when I looked up this very archaic reference, I thought the spelling of Marcia was actually Marsha, which is what I remember, and now I have no idea if my memory is just shitty, or if we have entered a Bernstein Bears/Berenstain Bears-Mandela Effect scenario.