Greetings from Oregon! Husbot and I are doing our month-long tour of America to visit family and friends. We’ll be on the west coast for a few weeks, and then over to the east coast for a bit, including Virginia and DC. In addition to re-acquainting myself with all of America’s ‘bigness’, I’m also taking some downtime, which means I’ll probably be writing more. And I’m definitely reading more, which is what inspired this post.
Also, I miss my cats, so you will get a cat photo for today’s post.
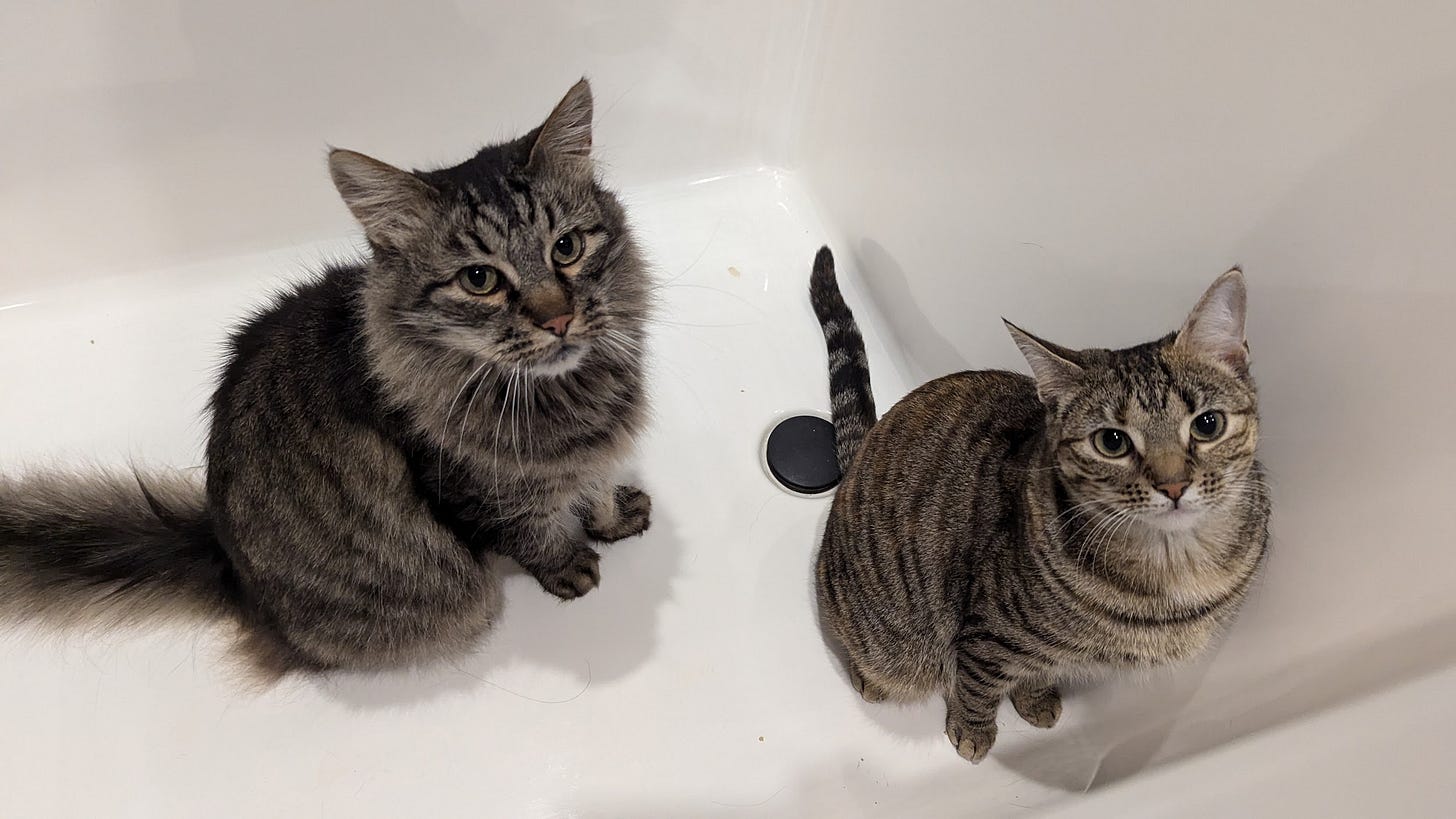
For Henry, it’s less about an Ouroboros of Shit / AI Model Collapse problem (obligatory link to that Nature study). Instead, he’s worried about something far more interesting. With an homage to researcher Alison Gopnik’s work, Farrell notes that AI is great at reproducing common patterns of culture that are already with us, but pretty lousy at exposing the novel or wierd, much less creating it:
That suggests a subtly different vision of the cultural downside of LLMs. As Alison Gopnik points out, LLMs are quite good at reproducing culture, but not so good at introducing cultural variation. One might go further. There is good reason to believe that these models are centripetal rather than centrifugal. On average, they create representations that tug in the direction of the dense masses at the center of culture, rather than towards the sparse fringe of weirdness and surprise scattered around the periphery.
Essentially, AI won’t eat itself, so much as spit out a bland middle that will in turn homogenize our culture, thoughts, science, and creativity. He continues:
What is common has cultural gravity. What is rare does not.
This has important implications, when combined with Gopnik’s thesis that large models are increasingly important engines of cultural reproduction. Such models will probably not subject human culture to the curse of recursion, in which noise feeds upon noise. Instead, they will parse human culture with a lossiness that skews, so that central aspects of that culture are accentuated, and sparser aspects disappear in translation. The thing about large models is that they tend to select for features that are common and against those that are counter, original, spare, strange. Instead of focusing on the gubbisher - a universally hungry agent of entropy and decay - one should emphasize the fact that it will disappear some aspects of culture more quickly than others.
And I think this is a far more interesting question to ask in the great AI debate (far better than the AI is shit/AI is amazing debate that I see on LinkedIn every damn day). More importantly, I agree with him.
But me agreeing with a random Substack guy is boring, and so, in pursuit of my inner contrarian asshole, I want to riff on a further point he made that I don’t agree with.
Are LLMs Missing Insights? Probably! But so are Most Humans
If AI and LLMs are bad because their outputs skew towards the common and miss the novel, Farrell argues that this may have dire consequences for cultural and scientific discovery. To illustrate that point, he shares a research paper which found that over half of the researchers interviewed in a user study preferred GPT-4-based “peer review” compared to human peer review, for some value of human peer. Here’s Farrell’s takeaway:
But what [the researchers] couldn’t easily test for - and what I think is the most crucial question - is whether AI reviews could identify and evaluate the features of the paper that were original and novel. I would lay a lot of money that AI reviews are much worse at this even than human reviewers. Such features are likely to be invisible to them, for much the same reason that Notebook LM had a harder time spotting the more unconventional claims of this newsletter.
He’s probably right here—LLNs are almost certainly worse at spotting novel, surprising, flawed, fraudulent, or biased observations and conclusions compared to a skilled and observant human reviewer. But is a large language model worse than the average human reviewer? Is it less observant than a generic peer who may be rushed, tired, biased towards conspiracy theories, indifferent to the subject matter, or hold petty grievances against the authors? Is ChatGPT better or worse than a human reviewer who sees only the final product and not the underlying data that conclusion is based on?
over at Experimental History has a fantastic post discussing this very point.I think most people weirdly assume that ‘peer review’ means substantive or quality review. Sometimes, its little more than someone saying ‘Looks Good to Me (LGTM)’ and hitting the approve button (or whatever).
My husband, aka, Husbot regularly complains about this problem when he receives code reviews approved moments after submitting a code change. Clearly, his peers aren’t speed-reading and providing deep insight on his mistakes or offering concrete improvements to his code. They’re clicking Accept and moving on to do the same for other requests. He derisively refers to these people as ‘LGTM bots’.
And this measure of mediocrity doesn’t end with academic papers and computer code. I’d hazard that this carries over to most other knowledge-based fields as well. The average human is, well, average at issue spotting, writing, and formulating complex, novel ideas, much less identifying gaps or inconsistencies in another person’s work. And most of us have enough stuff going on in our lives that we’re not going to stop and critically assess someone else’s work product unless a lot of money is riding on perfection. And sometimes, not even then.
FFS, we can’t even spot spelling errors half the time.1
Given that this is the reality of our world, it seems wrong to compare LLM review quality against some idealized best-case human review standard. It’s fair to say that LLMs suck against the best humans. But, if the option is an LLM review versus the average human’s middling attempt (or worse, no review at all), how can an LLM review actually be worse?
If the LLM can at least catch some errors or dubious conclusions, even if not the most surprising or novel ones, is that bad? What if an LLM catches the boring, obvious things (like spellcheck does), and allows human reviewers to prioritize assessing the novel. Wouldn’t that be better than what we have today?
Look, it’s pretty obvious that in some cases, LLMs are making people lazy, and this is a bad thing. And maybe we will continue to slide, Idiocracy-like towards a stupider, blander, less weird cultural/scientific homogeneity. I have no idea—I’m only a Privacy Cassandra, not a cultural one.
But I will hazard an observation: if we do slouch towards a cultural and/or scientific sameness, it’s not going to be because of ChatGPT. Or it won’t primarily be due to ChatGPT. If content curves inwards and becomes boring, it’s going to be because we’re all too focused on trying to keep the world from figuratively and literally burning, and not actively seeking out the novel.
IMHO, it’s far more likely to be the result of TikTok, Twitter, and the New York Times rather than AI-generated slop. The AI-generated stuff is dumb and obvious, whereas we humans have been creating shit content for millennia.
That said, I do think it would be extremely helpful if AI-based reviews (of any kind) had a helpful ‘Bot reviewed’ author label attached.
PS: I did run this article through ChatGPT and it gave me a few helpful suggestions around formatting and an idea for the title.
A free paid subscription to anyone who identifies the three mistakes I left behind in this post.